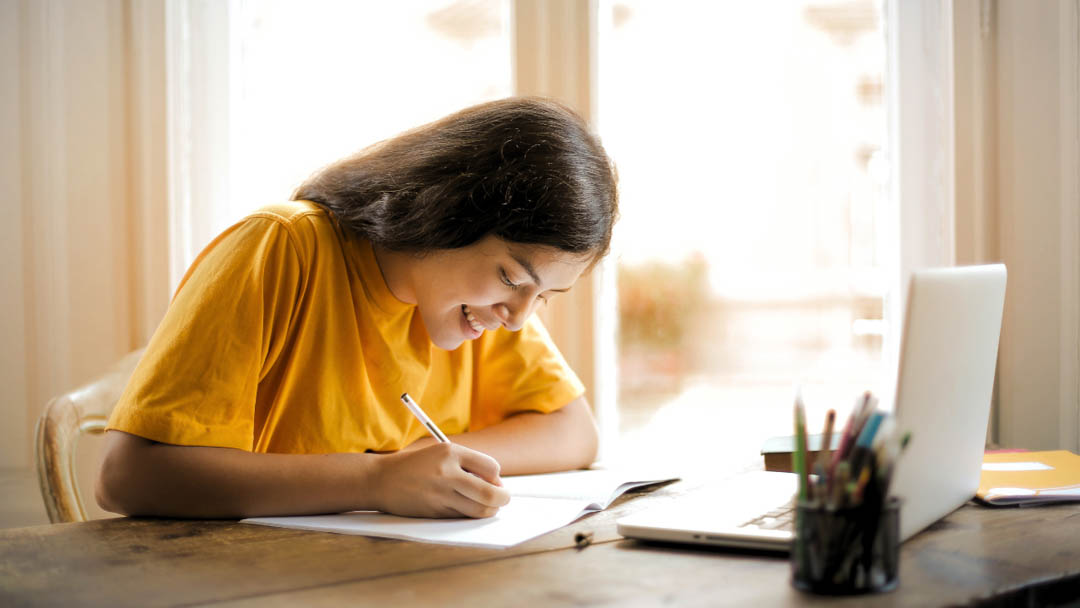
How to Transfer Credits and Maximize What You’ve Already Done
Thinking about transferring your credits, getting recognition for your work experience, or finishing your degree faster? We’re here to help make it happen. Whatever your path looks like, you’re not alone—we’ll be with you every step of the way.